Author: Neil Harrington
For decades, economists, researchers, and technologists have discussed the varying degrees to which automation will affect labor markets. Some observers conclude that automation will replace the need for human laborers, leading to widespread unemployment. Other observers say technological advancements are more likely to alter the tasks workers devote their time to, and, in other cases, shift workers into new occupations. This article builds on previous work examining the impacts of automation by applying estimates of occupational automation exposure to North Carolina’s labor market. The findings from this analysis suggest North Carolina’s labor market is slightly more exposed than the nation. The analysis also finds higher education can insulate workers from automation by giving them access to jobs with more uniquely human tasks and work contexts. While not predictive, this analysis should caution workers, employers, policy makers, and others about the occupations most likely to change due to technological advancement.
While threats are real, some previous research overstated automation impacts
Much of the focus around the risk automation poses to the national and regional economies involves projections of widespread destruction of jobs from continued technological progress. For example, a seminal paper in 2013 on automation’s impact on the United States labor force predicted that about half of total employment could disappear in coming decades due to advances in artificial intelligence (AI) and computerization. A few years later, a tech entrepreneur claimed automation would eliminate 80 to 90 percent of jobs over the next 10 to 15 years. Other analyses suggest automation will result in job losses by eliminating demand for occupations heavy in physical and routine skills, a more reasonable conclusion.
While automation may lead to the destruction of some jobs in our current economy, technological progress is more likely to cause occupational disruption. In some cases, technology can substitute for human labor. In 1900, for example, the agricultural industry employed 40 percent of United States workers, but advancements in farming technology contributed to a steep decline in agricultural employment over the next century, dropping to just 2 percent of total national employment by 2010. But technology can also improve productivity by complementing human labor and reducing the amount of time people spend on physical and routine tasks.
The advent of automated teller machines (ATMs) offers a good example of technological advancements disrupting rather than destroying jobs. As the name suggests, ATMs performed many of the typical tasks of bank tellers, such as fulfilling cash withdrawals and deposits. Following the conventional automation logic, ATMs substitution for traditional human labor should have eliminated many banks’ teller jobs. However, the number of bank tellers in the United States continued to gradually increase as more machines were installed across the country. The cost savings ATMs delivered to banks—combined with regulatory policy changes—allowed companies to open additional branches, increasing the demand for tellers. While ATMs took on more of the automatable tasks, tellers were able to focus more energy on more uniquely human tasks like interpersonal relationships with customers.
The ATM-bank teller anecdote previews a more likely automation scenario than widespread destruction of jobs. Machines might continue substituting for humans in more physical and routine tasks, but this would increasingly shift humans’ workplace responsibilities toward cognitive and nonroutine tasks that AI is less equipped to handle. Automation may reduce demand for certain occupations—as was the case for agricultural occupations as farming technology modernized—but that presents opportunities for workforce and education policy to equip people with skills and credentials to change occupations.
Occupations in North Carolina face slightly more exposure to automation disruptions than the United States
When viewed as more likely to disrupt employment patterns than outright destroy jobs, automation seems far less apocalyptic. The Labor Market Information Institute’s (LMI Institute) new automation exposure score frames automation’s threat in this way. LMI Institute researchers use O*NET data on occupational attributes to compute an automation exposure score for nearly every BLS-defined occupation.1 This analysis estimates North Carolina’s exposure to automation by applying LMI Institute’s automation exposure scores to the state’s occupational employment distribution.
North Carolina’s occupational employment mix tends toward risk categories more exposed to automation related disruptions. As Figure 1 shows, North Carolina has slightly higher shares of total employment in risk categories seven through nine than the United States and a slightly lower concentration of employment in categories less exposed to automation-related disruption. While there are differences between state and national automation exposure, the employment shares are not drastically different. Furthermore, the percentage of North Carolina’s total employment in the categories least exposed to automation (one and two) is more than double the share in the most exposed categories (nine and 10). Table 1 below presents the ten occupations in North Carolina most exposed to automation, according to the LMI Institute data.
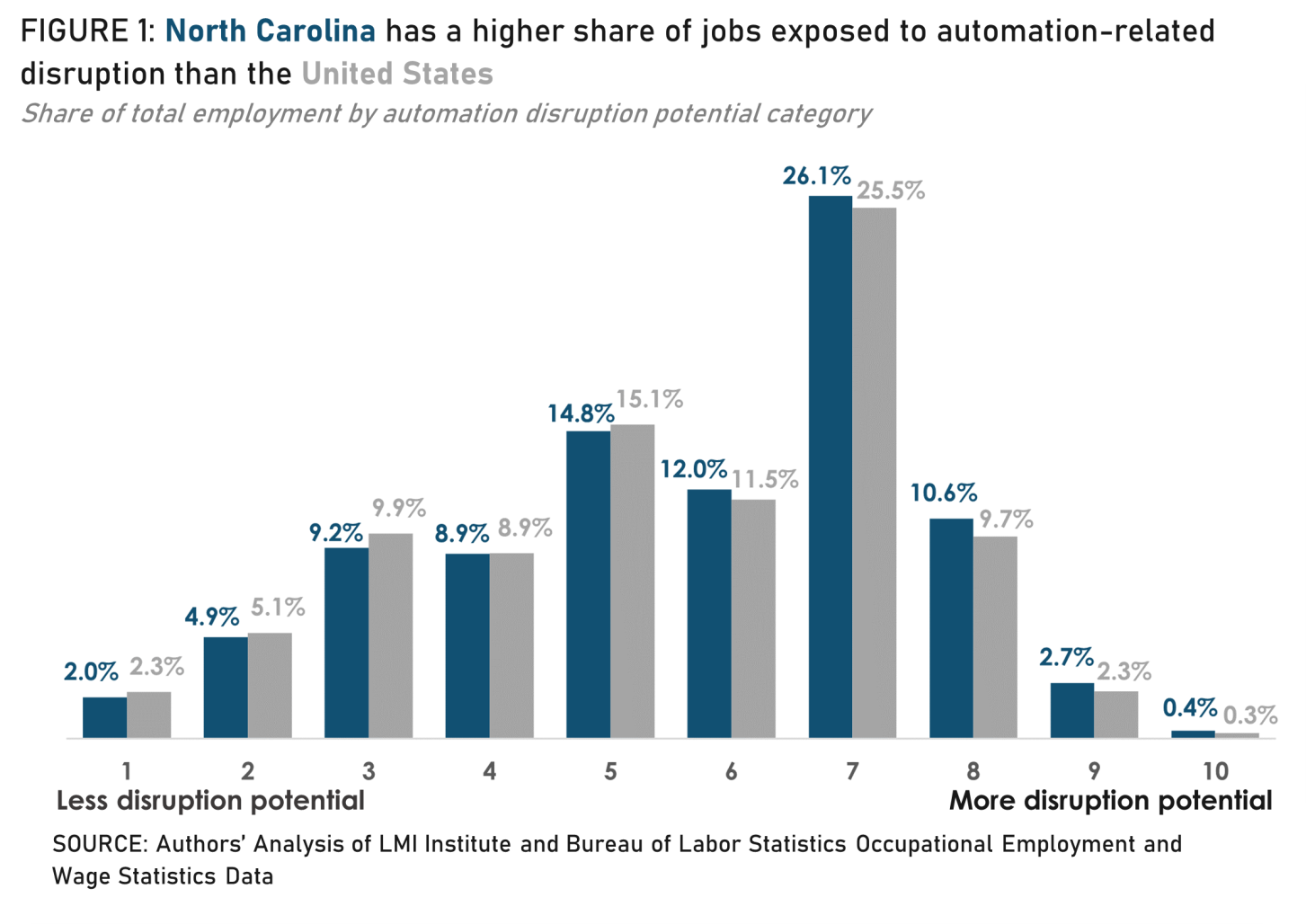
While North Carolina’s labor market is slightly more exposed to potential automation disruption than the nation, it is in no way predictive. This analysis—and LMI Institute’s underlying data—does not attempt to discern how occupations might change, when they might change, or whether disruption is guaranteed. Instead, this analysis should caution workers, employers, workforce professionals, policy makers, and education leaders about the occupations most likely to change due to technological advancements. People in these roles might anticipate disruption in the most exposed jobs by expanding credential programs in the least exposed occupations; pursuing education or training necessary to move out of an exposed job and into a less exposed job; or otherwise taking action to minimize automation’s labor market disruption potential.
Occupations with higher education requirements are less exposed to automation employment disruptions
As Figure 2 shows, average automation exposure scores are lower among occupations with higher typical minimum educational attainment requirements. This insulation from automation related employment loss is similar to the resiliency higher education offers during economic downturns. Higher education provides individuals with more employment options relatively safe from potential disruption and the versatility to change into a job less affected by automation if their current job is disrupted. For example, only about 2 percent of employment in occupations requiring a bachelor’s degree or higher faces moderately high or high potential for automation disruption, compared to about 61 percent of employment in occupations requiring just a high school diploma or less (see Figure 3).2
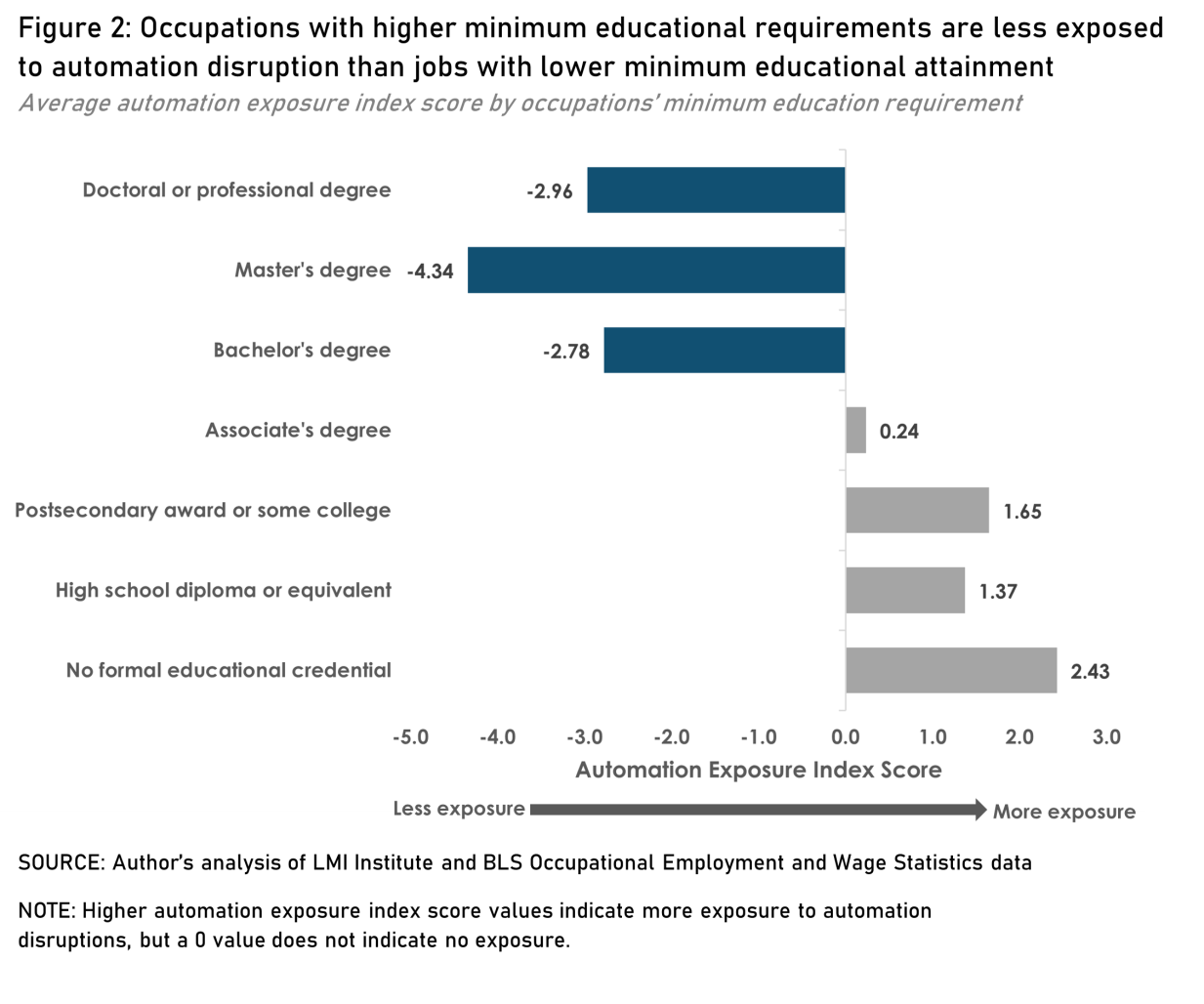
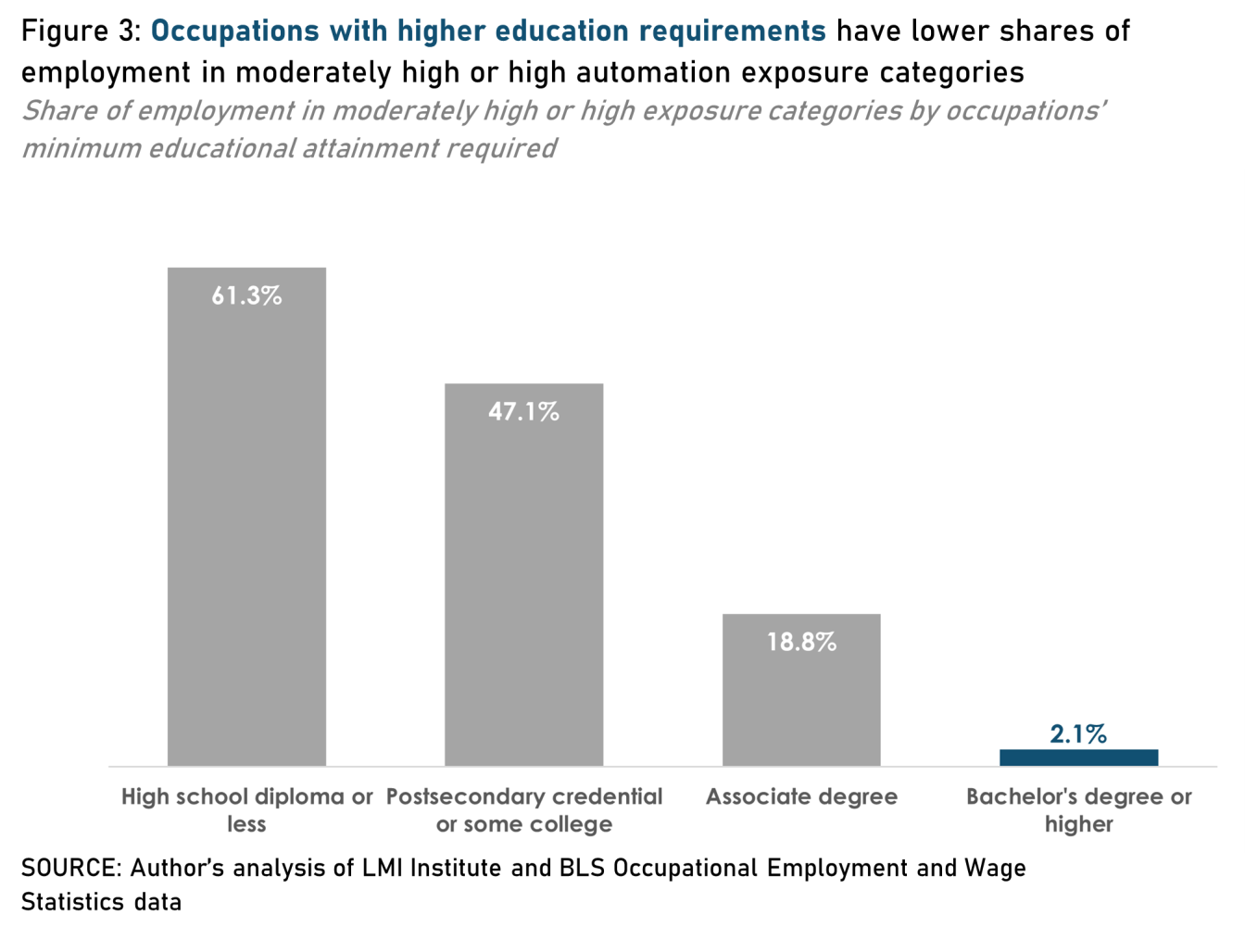
The relationship between occupations requiring low levels of education and greater exposure to automation employment disruption emphasizes the importance of educational attainment for the future of work. As this analysis indicates, higher educational attainment clearly provides some security from automation disruption or outright job loss. Higher education typically sharpens abilities and other skillsets that separate human labor from machines. As technological progress chugs along, occupations with low education requirements could experience more disruption, stressing the importance of assisting people in obtaining the skills and credentials needed for jobs less affected by automation.
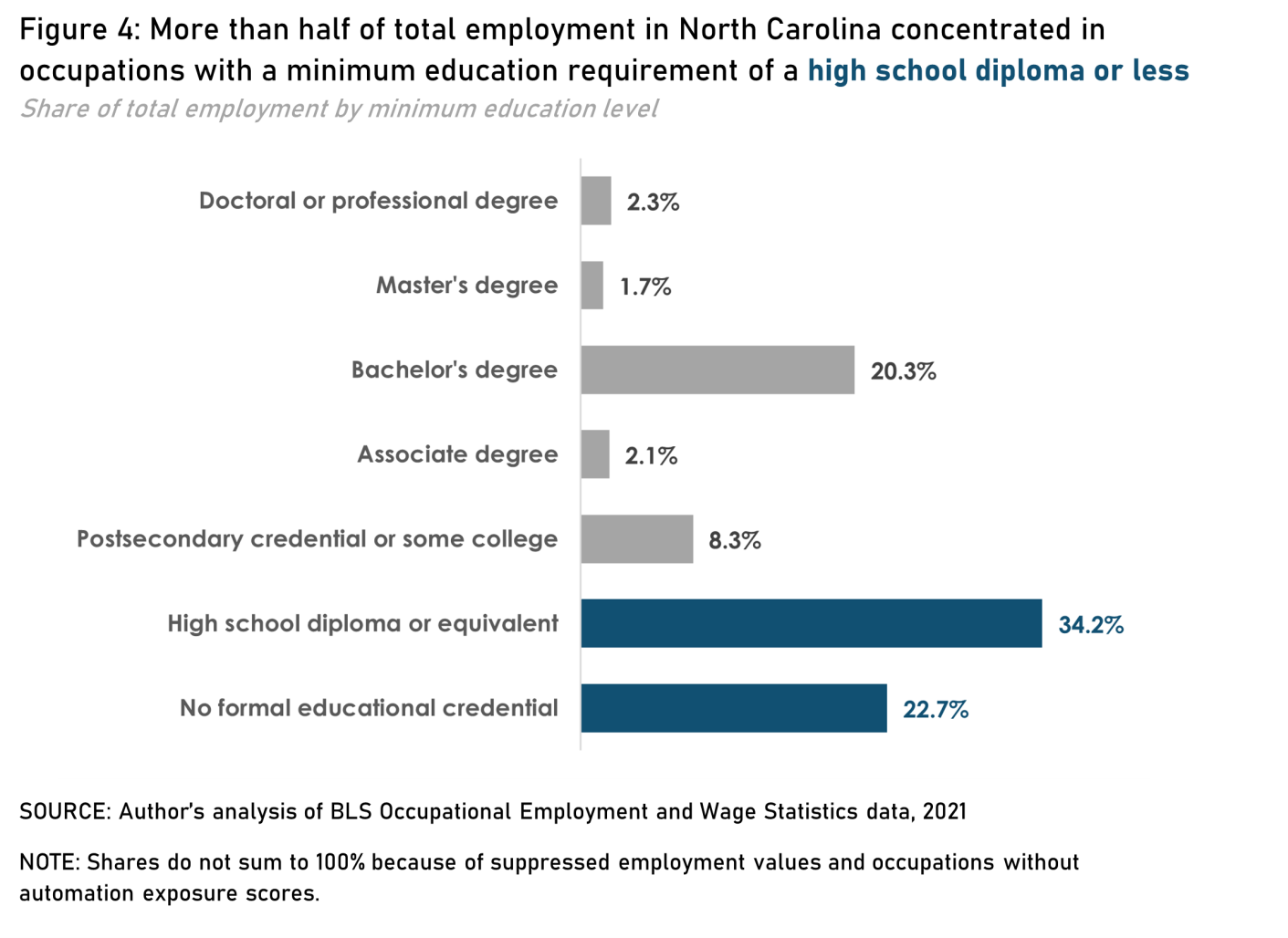
North Carolina’s share of total occupational employment by typical minimum education level further fuels automation’s disruption potential. As shown in Figure 4, occupations requiring a high school diploma or less accounted for about 57 percent of the state’s total employment in 2021. As mentioned previously, this does not mean that automation will eliminate or drastically disrupt all—or even most—of these jobs. But these data do indicate many people in North Carolina work in occupations with tasks that are more susceptible to disruption.
Alternative measures of automation related employment risks and their methodologies
This analysis joins other work that frames automation’s labor market threat as disruption rather than destruction. Researchers at the Information Technology and Innovation Foundation (ITIF) constructed an automation risk gauge for the more than 800 occupations defined by the U.S. Bureau of Labor Statistics (BLS). Their analysis found that only about eight percent of occupations in the United States are at high risk of automation, while 15 percent of occupations face a “low” risk from automation-related job loss. Other states have used ITIF’s risk categories to assess regional labor markets’ susceptibility to automation employment disruption and arrived at similar conclusions. For example, Minnesota and Ohio have relatively few occupations in the “high risk” category—7.8 and 7.6 percent of total employment, respectively—but nearly double these shares in the lowest automation risk category (16.2 and 14.2 percent, respectively).
The LMI Institute’s occupational automation exposure scores used in this analysis takes a similar approach. LMI Institute determines an occupation’s exposure to automation related job losses based on O*NET data on occupational attributes. These data consider abilities needed for an occupation’s work activities and work contexts, among other factors. LMI Institute takes these occupational attributes and weights them by their importance or time devoted to each within an occupation and then ranks occupations on a scale from 1 (least exposed) to 10 (most exposed).
The LMI Institute’s data builds on ITIF’s previous work. Both analyses present a different perspective than the traditional view on the threat automation poses to US labor markets, framing risks from technological progress more as exposure to employment disruption rather than widespread destruction of jobs. However, the two bodies of work differ in methodological approaches. ITIF manually assessed each BLS-defined occupation’s automatability based on their educational requirements, qualitative assessments of job scope, and the presence of technologies that can substitute for human labor. LMI Institute advances ITIF’s work by incorporating the sixteen O*NET work characteristics most associated with occupational employment changes from automation. Researchers calculate numeric scores for these characteristics based on their relative importance to an occupation and average across five categories.3 LMI Institute’s more rigorous quantitative approach to estimating automation exposure motivated its use over ITIF’s in this analysis.
This is the first in a series of articles examining the impacts of automation and how automation research can inform understandings of current and future labor market demand. Future articles in the series will apply automation exposure indexes at the industry level in North Carolina, explore what automation exposure means for economic and workforce development policy, examine regional impacts of automation exposure, and more.
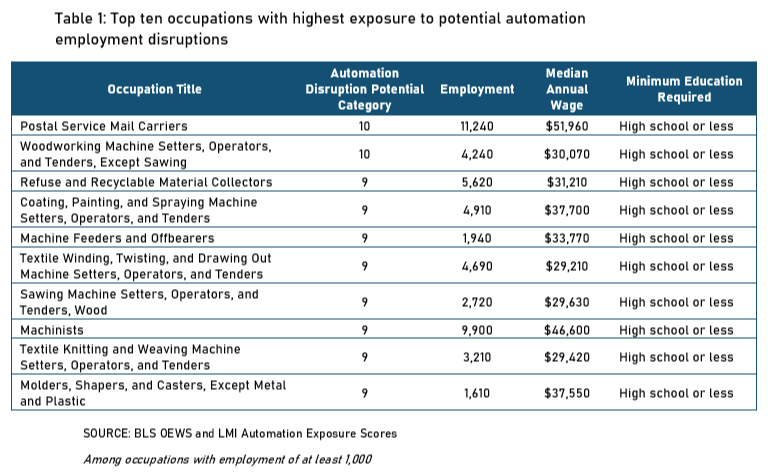
1The last section of this article presents additional details on LMI Institute’s methodology.
2Interestingly, the only bachelor’s degree or higher occupation in the high exposure category is Airline Pilots, Copilots, and Flight Engineers. This illustrates the limitations of an automation index and automation’s employment impacts in general. While the typical tasks done by pilots may be automatable, it is unlikely many people will purchase airline tickets for flights directed by a robot.
3The five categories are Cognitive Analytical, Cognitive Interpersonal, Routine Cognitive, Routine Manual, and Nonroutine Manual. The scores for categories making an occupation less exposed to automation (Cognitive Analytical and Cognitive Interpersonal) are then subtracted from the categories more exposed to automation to arrive at an occupation’s automation exposure index score.